Twenty years ago, my paternal grandfather passed away. While we were all dealing with the grief of the situation and planning his funeral, my mother handed me a stack of what seemed like a million old family photographs.
She, being a genealogy buff, instructed me to “Find some good photographs of your grandfather” and “Digitize every photo using this fancy scanner”. She also told me to label every person who appeared in each photo, along with where it was taken and what was going on in the photo. Some of the photos dated back to when I was a five-year-old kid running around with my cousins on a hot summer’s day. Others were only a few years old, taken when we were visiting Grandma and Grandpa and eating homemade chicken noodles and mashed potatoes that my grandma made from scratch.
At the time, going through these photos was a very emotional and tedious task. Off and on over the course of the week, I sat there slowly putting a photo in the scanner one-by-one, hitting the scan button, and writing in a journal all the information I could extract out of the photo. I know I was probably making a few mistakes along the way, but I didn’t care, I just wanted this long process to end.
Today, every single photograph taken is natively stored in a digital form in the camera and in many cases, stored online immediately after it is taken. Throughout the Internet, we all have access to millions of photographs of people, our friends, pets, food, buildings, flowers, and landscapes. These are all logged automatically using artificial intelligence. I can open my photos app on my smart phone, select a photo and it automatically shows me all the photos I have that have the same person in it. I can simply search for “car” and find all the pictures of my old red sports car that I used to drive to university every day. I can digitally erase the photobomber out of the picture that I don’t want in that otherwise perfect shot I took. It’s quite amazing that technology exists so readily, right in our hands. You don’t need a Ph.D. in computer vision to be able to do this sort of work, you just need the right application to help you out.
Here at Solv3d, our customers collect thousands upon thousands of miles (or kilometers) of LiDAR, orthomosaics, and panoramic images every year. They are mapping the world and creating a digitized version of it. This digitalization requires them to identify areas (or objects) of interest, such as street signs.
For example, a municipality may want to quickly identify the location of all their stop signs and yield signs, and then cross reference this against a list of dates the signs were installed. They may need to use this information for maintenance; a maintenance manager could use this information to estimate costs to upgrade all these signs throughout the entire city boundary.
We set out to provide a solution that will allow our customers to cost effectively identify objects of interest in their datasets in a timely manner. We knew that whatever we came up with had to produce results consistent between datasets. We also set ourselves a goal to develop a solution that was both secure (the data and the model had to remain local to the user) and flexible (the user should be able to identify whatever is important to them, not what we thought was important). Our solution is a deep learning-based tool that our customers can use to produce their own model and then use it to identify objects of interest in their data.
The process is simple and very flexible. Users load a recent set of panoramic images into Engine that they then use to train a model. They do this by using Engine to draw polygons around 10 to 20 examples of the object they are interested in, such as a stop sign or a yield sign [Figure 1].
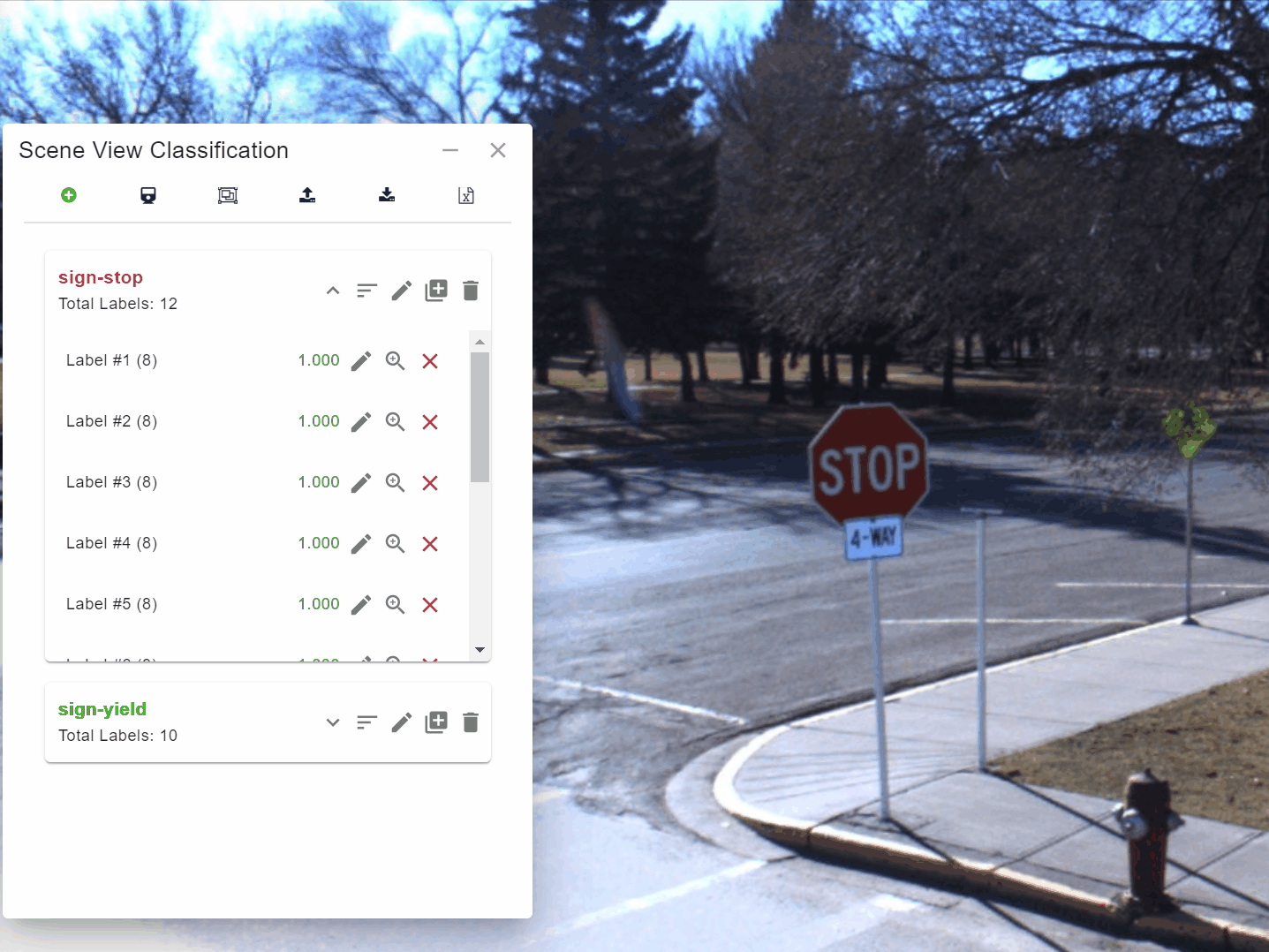
They then press a button to have Engine “teach” the model about the example. As Engine is teaching the model, they can monitor the accuracy of the model as it works through its learning process [Figure 2].
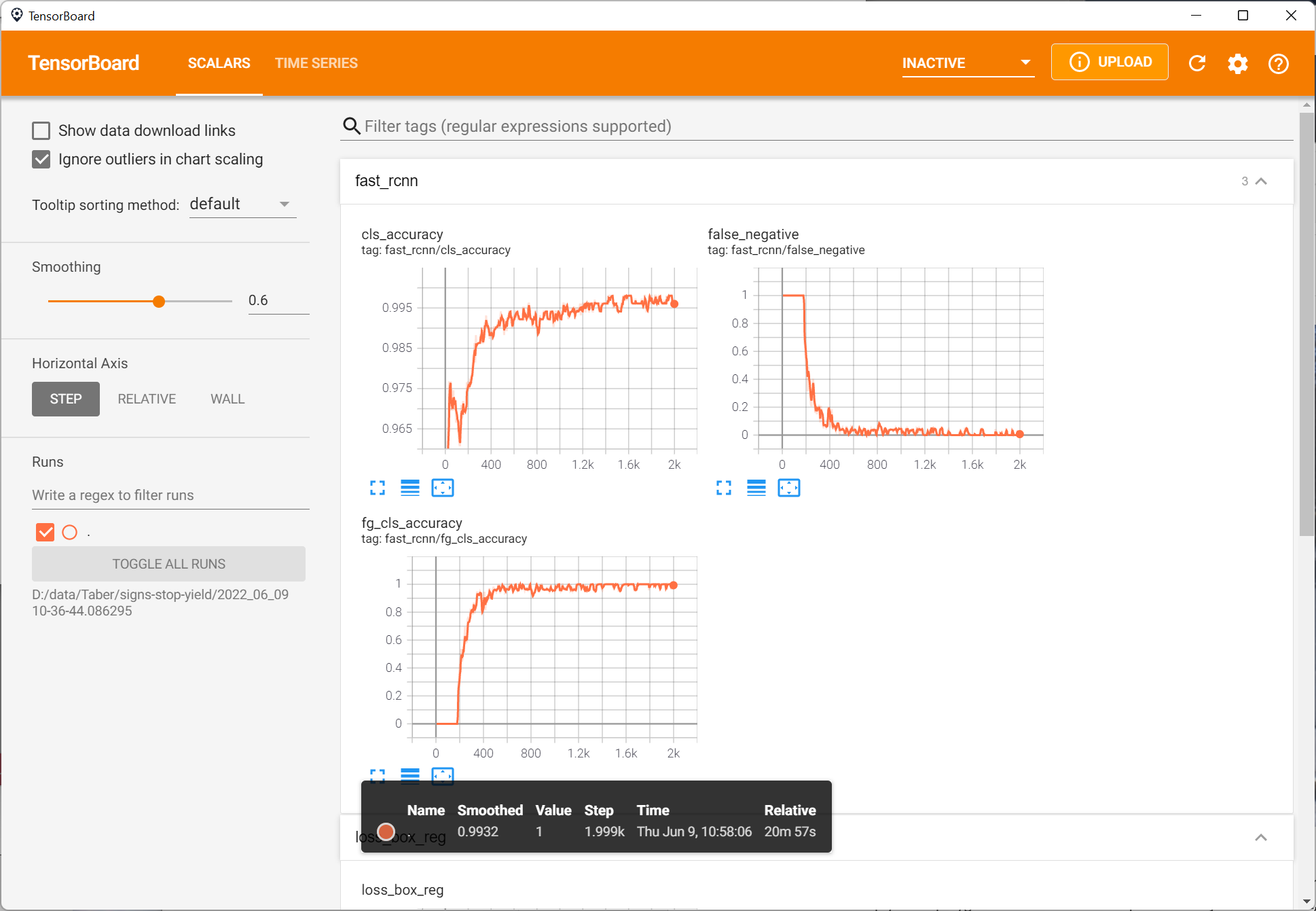
When Engine has finished training the model, users then use it to apply the model to their data. Engine will show the results on the screen.
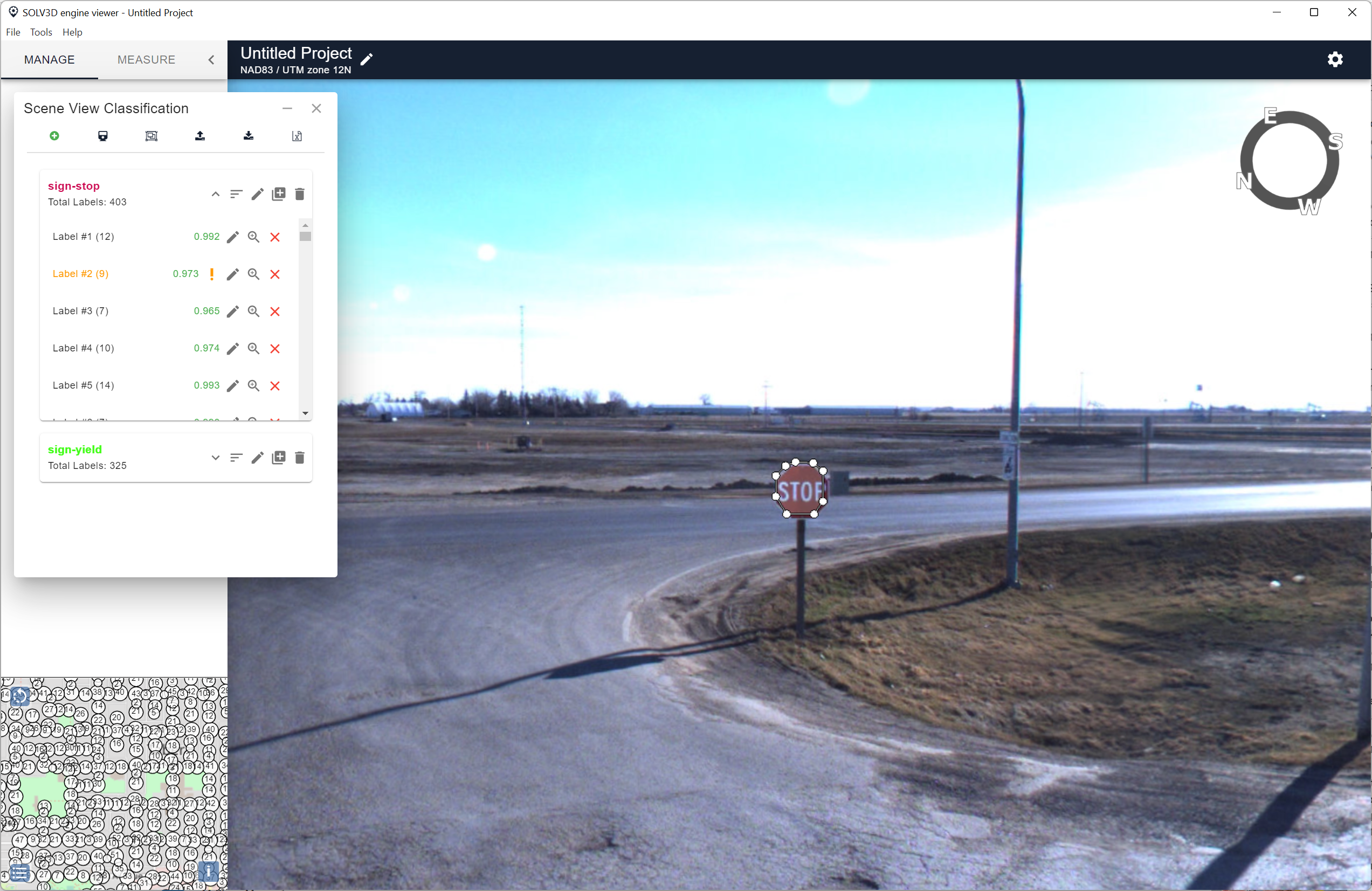
Finally, Engine can export the results, including an estimate of the locations of each object, in multiple formats such as CSV. This allows users to share the results with others, or use the results in other applications such as SOLV3D Encompass or Esri ArcGIS. Figure 4 shows results inside of Encompass.
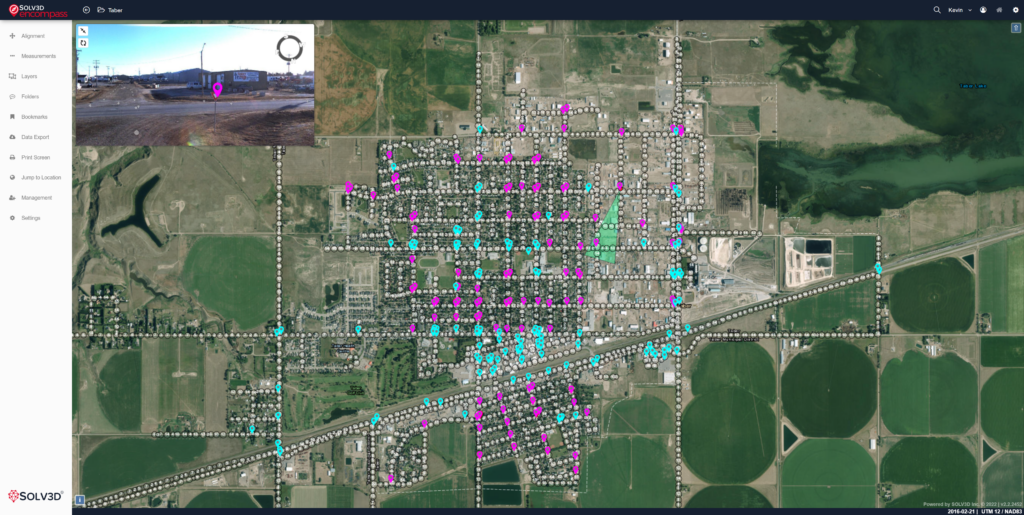
Once you have trained the model, you can use that model any time on other data to identify the same object of interest. The model is very cost effective to use on subsequent datasets, and much faster when compared to a manual process.
Because Engine is a local application, you have complete control over your data and your model. Both the model and your data stay with you. The model is yours and is not shared with anyone else, which means you can use your data strategically by training it to identify unique items that your competitors may not have data for.
Because the model is computer based, the results are consistent, and Engine will provide you with a statistical estimation of its effectiveness at identifying the objects – something difficult (if not impossible) to do with a manual process.
Do I wish I had this technology 20 years ago when I was cataloging all those photographs for my mother?
I sure do!
What I find even more amazing is that this technology is only a stepping stone towards what is yet to come with automatic analysis of geospatial datasets. In the very near future, using the combination of imagery and point cloud datasets, we plan to deliver more value by aggregation of results of different datasets to improve the accuracy of detection and to automate workflows even further.
We developed an intensive, hands-on workshop to introduce you to deep learning and how it can be applied to geospatial data like imagery. To learn more, please attend our workshop.
Additionally, if you would like more information on how Engine can help identify items you need to find in your data, contact us at sales@solv3d.com.